Meta和谷歌宣布推出新版自研人工智能芯片
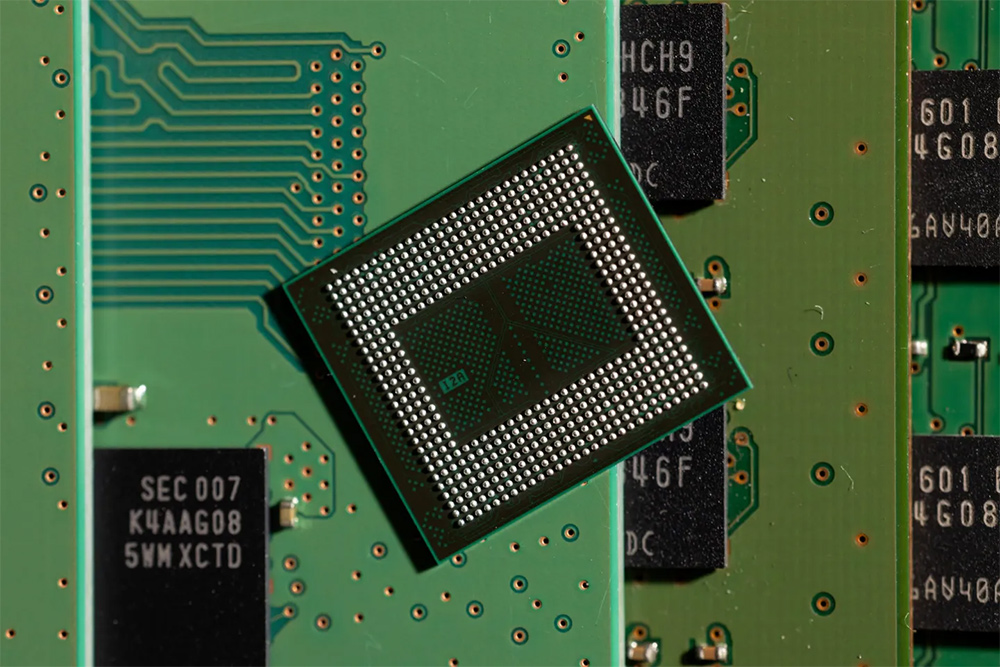
就在谷歌宣布推出Axion人工智能芯片之后,Meta刚刚宣布将进一步进军人工智能芯片竞赛。这两家公司都宣称,它们的新型半导体模型是开发人工智能平台的关键,也是它们和科技行业其他公司一直依赖的英伟达芯片的替代品,能够为人工智能数据中心提供动力。
硬件正在成为人工智能的关键增长领域。对于拥有资金和人才的大型科技公司来说,开发自研芯片有助于减少对英伟达和英特尔(Intel)等外部设计商的依赖,同时还允许公司专门根据自己的人工智能模型定制硬件,从而提高性能并节省能源成本。
谷歌和Meta刚刚宣布推出的这些自研人工智能芯片,对英伟达在人工智能硬件市场的主导地位构成了第一个真正的挑战。英伟达控制着超过90%的人工智能芯片市场,对其行业领先的半导体的需求只增不减。但如果英伟达最大的客户转而开始生产自己的芯片,那么其自年初以来飙升了87%的股价可能会受到影响。
科技咨询公司Omdia的分析师爱德华·威尔福德(Edward Wilford)在接受《财富》杂志采访时表示:“从Meta的角度来看……这为它们提供了与英伟达讨价还价的工具。这让英伟达知道,它们不是排他性的,而且还有其他选择。它们制造的硬件针对其正在开发的人工智能进行了优化。”
为什么人工智能需要新芯片?
人工智能模型需要大量的算力,因为需要大量的数据来训练背后的大型语言模型。传统的计算机芯片根本无法处理构建人工智能模型的数万亿个数据点,这催生了人工智能专用计算机芯片市场,这些芯片通常被称为“尖端”芯片,因为它们是市场上功能最强大的设备。
半导体巨头英伟达主导了这一新兴市场:英伟达价值3万美元的旗舰人工智能芯片的等待名单长达数月之久,需求推动该公司股价在过去六个月上涨了近90%。
竞争对手芯片制造商英特尔也在努力保持竞争力。它刚刚发布了Gaudi 3人工智能芯片,与英伟达展开直接竞争。上至谷歌和微软,下至小型初创企业,人工智能开发者都在争夺稀缺的人工智能芯片,但受到制造能力的限制。
为什么科技公司开始制造自己的芯片?
英伟达和英特尔只能生产有限数量的芯片,因为它们和业内其他公司都依赖中国台湾的制造商台积电(TSMC)来实际组装芯片设计。由于只有一家制造商参与其中,这些尖端芯片的制造周期长达数月。这是导致人工智能领域的主要参与者,如谷歌和Meta,自行设计芯片的一大关键因素。
咨询公司弗雷斯特市场咨询(Forrester)的高级分析师阿尔文·阮(Alvin Nguyen)告诉《财富》杂志,谷歌、Meta和亚马逊等公司设计的芯片不会像英伟达的顶级产品那样功能强大,但可能会在速度方面使这些公司受益。他说,它们将能够在专业化程度更低的装配线上生产这些产品,等待时间更短。
阮说:“如果你有产品性能差10%,但现在就能买到的东西,我每天都会买入。”
即使Meta和谷歌正在开发的原生人工智能芯片不如英伟达的尖端人工智能芯片功能强大,但它们可以更好地针对公司特定的人工智能平台进行定制。阮表示,为公司自己的人工智能平台设计的自研芯片可以通过消除不必要的功能来提高效率并节省成本。
阮说:“这就像买车一样。好吧,你需要自动变速箱。但你需要真皮座椅,还是加热按摩座椅呢?”
Meta发言人梅兰妮·罗伊在给《财富》杂志的一封电子邮件中写道:“对我们来说,这样做的好处是,我们可以打造一款能够更有效地处理特定工作负载的芯片。”
英伟达的顶级芯片每块售价约为2.5万美元。它们是极其强大的工具,而且设计用于广泛的应用,从训练人工智能聊天机器人到生成图像,再到开发推荐算法,比如TikTok和Instagram上的算法。这意味着功能稍弱,但更有针对性的芯片可能更适合Meta这样的公司。Meta在人工智能方面的投资主要是用于其推荐算法,而不是面向消费者的聊天机器人。
晨星研究公司(Morningstar)股票研究主管布莱恩·科莱洛(Brian Colello)告诉《财富》杂志:“英伟达的图形处理器(GPU)在人工智能数据中心中表现出色,但它们是通用型的。在某些工作负载和某些模型中,定制芯片可能会更好。”
万亿美元的问题
阮表示,更专业的自研芯片可以凭借其集成到现有数据中心的能力带来额外的好处。英伟达的芯片耗电量大、发热量高、噪音大,以至于科技公司可能被迫重新设计或迁移其数据中心,以集成隔音和液冷系统。功能较弱的原生芯片能耗低、发热量少,可以解决这个问题。
Meta和谷歌开发的人工智能芯片是长期赌注。阮估计,这些芯片的开发大约需要一年半时间,而大规模应用可能还需要数月时间。在可预见的未来,整个人工智能世界仍将在很大程度上依赖英伟达(其次是英特尔,依赖程度相对较小)来满足其计算硬件需求。事实上,马克·扎克伯格最近宣布,Meta有望在今年年底前拥有35万块英伟达芯片(届时该公司将在芯片上投入约180亿美元)。但从外包算力转向自研芯片设计,可能会打破英伟达的垄断。
科莱洛表示:“这些自研芯片的威胁是英伟达估值达万亿美元。如果这些自研芯片显著减少了对英伟达的依赖,那么英伟达的股票可能会因此下跌。这一事态发展并不令人意外,但未来几年的执行情况是我们关注的关键估值问题。”(财富中文网)
译者:中慧言-王芳
就在谷歌宣布推出Axion人工智能芯片之后,Meta刚刚宣布将进一步进军人工智能芯片竞赛。这两家公司都宣称,它们的新型半导体模型是开发人工智能平台的关键,也是它们和科技行业其他公司一直依赖的英伟达芯片的替代品,能够为人工智能数据中心提供动力。
硬件正在成为人工智能的关键增长领域。对于拥有资金和人才的大型科技公司来说,开发自研芯片有助于减少对英伟达和英特尔(Intel)等外部设计商的依赖,同时还允许公司专门根据自己的人工智能模型定制硬件,从而提高性能并节省能源成本。
谷歌和Meta刚刚宣布推出的这些自研人工智能芯片,对英伟达在人工智能硬件市场的主导地位构成了第一个真正的挑战。英伟达控制着超过90%的人工智能芯片市场,对其行业领先的半导体的需求只增不减。但如果英伟达最大的客户转而开始生产自己的芯片,那么其自年初以来飙升了87%的股价可能会受到影响。
科技咨询公司Omdia的分析师爱德华·威尔福德(Edward Wilford)在接受《财富》杂志采访时表示:“从Meta的角度来看……这为它们提供了与英伟达讨价还价的工具。这让英伟达知道,它们不是排他性的,而且还有其他选择。它们制造的硬件针对其正在开发的人工智能进行了优化。”
为什么人工智能需要新芯片?
人工智能模型需要大量的算力,因为需要大量的数据来训练背后的大型语言模型。传统的计算机芯片根本无法处理构建人工智能模型的数万亿个数据点,这催生了人工智能专用计算机芯片市场,这些芯片通常被称为“尖端”芯片,因为它们是市场上功能最强大的设备。
半导体巨头英伟达主导了这一新兴市场:英伟达价值3万美元的旗舰人工智能芯片的等待名单长达数月之久,需求推动该公司股价在过去六个月上涨了近90%。
竞争对手芯片制造商英特尔也在努力保持竞争力。它刚刚发布了Gaudi 3人工智能芯片,与英伟达展开直接竞争。上至谷歌和微软,下至小型初创企业,人工智能开发者都在争夺稀缺的人工智能芯片,但受到制造能力的限制。
为什么科技公司开始制造自己的芯片?
英伟达和英特尔只能生产有限数量的芯片,因为它们和业内其他公司都依赖中国台湾的制造商台积电(TSMC)来实际组装芯片设计。由于只有一家制造商参与其中,这些尖端芯片的制造周期长达数月。这是导致人工智能领域的主要参与者,如谷歌和Meta,自行设计芯片的一大关键因素。
咨询公司弗雷斯特市场咨询(Forrester)的高级分析师阿尔文·阮(Alvin Nguyen)告诉《财富》杂志,谷歌、Meta和亚马逊等公司设计的芯片不会像英伟达的顶级产品那样功能强大,但可能会在速度方面使这些公司受益。他说,它们将能够在专业化程度更低的装配线上生产这些产品,等待时间更短。
阮说:“如果你有产品性能差10%,但现在就能买到的东西,我每天都会买入。”
即使Meta和谷歌正在开发的原生人工智能芯片不如英伟达的尖端人工智能芯片功能强大,但它们可以更好地针对公司特定的人工智能平台进行定制。阮表示,为公司自己的人工智能平台设计的自研芯片可以通过消除不必要的功能来提高效率并节省成本。
阮说:“这就像买车一样。好吧,你需要自动变速箱。但你需要真皮座椅,还是加热按摩座椅呢?”
Meta发言人梅兰妮·罗伊在给《财富》杂志的一封电子邮件中写道:“对我们来说,这样做的好处是,我们可以打造一款能够更有效地处理特定工作负载的芯片。”
英伟达的顶级芯片每块售价约为2.5万美元。它们是极其强大的工具,而且设计用于广泛的应用,从训练人工智能聊天机器人到生成图像,再到开发推荐算法,比如TikTok和Instagram上的算法。这意味着功能稍弱,但更有针对性的芯片可能更适合Meta这样的公司。Meta在人工智能方面的投资主要是用于其推荐算法,而不是面向消费者的聊天机器人。
晨星研究公司(Morningstar)股票研究主管布莱恩·科莱洛(Brian Colello)告诉《财富》杂志:“英伟达的图形处理器(GPU)在人工智能数据中心中表现出色,但它们是通用型的。在某些工作负载和某些模型中,定制芯片可能会更好。”
万亿美元的问题
阮表示,更专业的自研芯片可以凭借其集成到现有数据中心的能力带来额外的好处。英伟达的芯片耗电量大、发热量高、噪音大,以至于科技公司可能被迫重新设计或迁移其数据中心,以集成隔音和液冷系统。功能较弱的原生芯片能耗低、发热量少,可以解决这个问题。
Meta和谷歌开发的人工智能芯片是长期赌注。阮估计,这些芯片的开发大约需要一年半时间,而大规模应用可能还需要数月时间。在可预见的未来,整个人工智能世界仍将在很大程度上依赖英伟达(其次是英特尔,依赖程度相对较小)来满足其计算硬件需求。事实上,马克·扎克伯格最近宣布,Meta有望在今年年底前拥有35万块英伟达芯片(届时该公司将在芯片上投入约180亿美元)。但从外包算力转向自研芯片设计,可能会打破英伟达的垄断。
科莱洛表示:“这些自研芯片的威胁是英伟达估值达万亿美元。如果这些自研芯片显著减少了对英伟达的依赖,那么英伟达的股票可能会因此下跌。这一事态发展并不令人意外,但未来几年的执行情况是我们关注的关键估值问题。”(财富中文网)
译者:中慧言-王芳
Meta just announced it’s pushing further into the AI chip race, coming right on the heels of Google’s own announcement of its Axion AI chip. Both companies are touting their new semiconductor models as key to the development of their AI platforms, and as alternatives to the Nvidia chips they—and the rest of the tech industry—have been relying on to power AI data centers.
Hardware is emerging as a key AI growth area. For Big Tech companies with the money and talent to do so, developing in-house chips helps reduce dependence on outside designers such as Nvidia and Intel while also allowing firms to tailor their hardware specifically to their own AI models, boosting performance and saving on energy costs.
These in-house AI chips that Google and Meta just announced pose one of the first real challenges to Nvidia’s dominant position in the AI hardware market. Nvidia controls more than 90% of the AI chips market, and demand for its industry-leading semiconductors is only increasing. But if Nvidia’s biggest customers start making their own chips instead, its soaring share price, up 87% since the start of the year, could suffer.
“From Meta’s point of view … it gives them a bargaining tool with Nvidia,” Edward Wilford, an analyst at tech consultancy Omdia, told Fortune. “It lets Nvidia know that they’re not exclusive, [and] that they have other options. It’s hardware optimized for the AI that they are developing.”
Why does AI need new chips?
AI models require massive amounts of computing power because of the huge amount of data required to train the large language models behind them. Conventional computer chips simply aren’t capable of processing the trillions of data points AI models are built upon, which has spawned a market for AI-specific computer chips, often called “cutting-edge” chips because they’re the most powerful devices on the market.
Semiconductor giant Nvidia has dominated this nascent market: The wait list for Nvidia’s $30,000 flagship AI chip is months long, and demand has pushed the firm’s share price up almost 90% in the past six months.
And rival chipmaker Intel is fighting to stay competitive. It just released its Gaudi 3 AI chip to compete directly with Nvidia. AI developers—from Google and Microsoft down to small startups—are all competing for scarce AI chips, limited by manufacturing capacity.
Why are tech companies starting to make their own chips?
Both Nvidia and Intel can produce only a limited number of chips because they and the rest of the industry rely on Taiwanese manufacturer TSMC to actually assemble their chip designs. With only one manufacturer solidly in the game, the manufacturing lead time for these cutting-edge chips is multiple months. That’s a key factor that led major players in the AI space, such as Google and Meta, to resort to designing their own chips. Alvin Nguyen, a senior analyst at consulting firm Forrester, told Fortune that chips designed by the likes of Google, Meta, and Amazon won’t be as powerful as Nvidia’s top-of-the-line offerings—but that could benefit the companies in terms of speed. They’ll be able to produce them on less specialized assembly lines with shorter wait times, he said.
“If you have something that’s 10% less powerful but you can get it now, I’m buying that every day,” Nguyen said.
Even if the native AI chips Meta and Google are developing are less powerful than Nvidia’s cutting-edge AI chips, they could be better tailored to the company’s specific AI platforms. Nguyen said that in-house chips designed for a company’s own AI platform could be more efficient and save on costs by eliminating unnecessary functions.
“It’s like buying a car. Okay, you need an automatic transmission. But do you need the leather seats, or the heated massage seats?” Nguyen said.
“The benefit for us is that we can build a chip that can handle our specific workloads more efficiently,” Melanie Roe, a Meta spokesperson, wrote in an email to Fortune.
Nvidia’s top-of-the-line chips sell for about $25,000 apiece. They’re extremely powerful tools, and they’re designed to be good at a wide range of applications, from training AI chatbots to generating images to developing recommendation algorithms such as the ones on TikTok and Instagram. That means a slightly less powerful, but more tailored chip could be a better fit for a company such as Meta, for example—which has invested in AI primarily for its recommendation algorithms, not consumer-facing chatbots.
“The Nvidia GPUs are excellent in AI data centers, but they are general purpose,” Brian Colello, equity research lead at Morningstar, told Fortune. “There are likely certain workloads and certain models where a custom chip might be even better.”
The trillion-dollar question
Nguyen said that more specialized in-house chips could have added benefits by virtue of their ability to integrate into existing data centers. Nvidia chips consume a lot of power, and they give off a lot of heat and noise—so much so that tech companies may be forced to redesign or move their data centers to integrate soundproofing and liquid cooling. Less powerful native chips, which consume less energy and release less heat, could solve that problem.
AI chips developed by Meta and Google are long-term bets. Nguyen estimated that these chips took roughly a year and a half to develop, and it’ll likely be months before they’re implemented at a large scale. For the foreseeable future, the entire AI world will continue to depend heavily on Nvidia (and, to a lesser extent, Intel) for its computing hardware needs. Indeed, Mark Zuckerberg recently announced that Meta was on track to own 350,000 Nvidia chips by the end of this year (the company’s set to spend around $18 billion on chips by then). But movement away from outsourcing computing power and toward native chip design could loosen Nvidia’s chokehold on the market.
“The trillion-dollar question for Nvidia’s valuation is the threat of these in-house chips,” Colello said. “If these in-house chips significantly reduce the reliance on Nvidia, there’s probably downside to Nvidia’s stock from here. This development is not surprising, but the execution of it over the next few years is the key valuation question in our mind.”