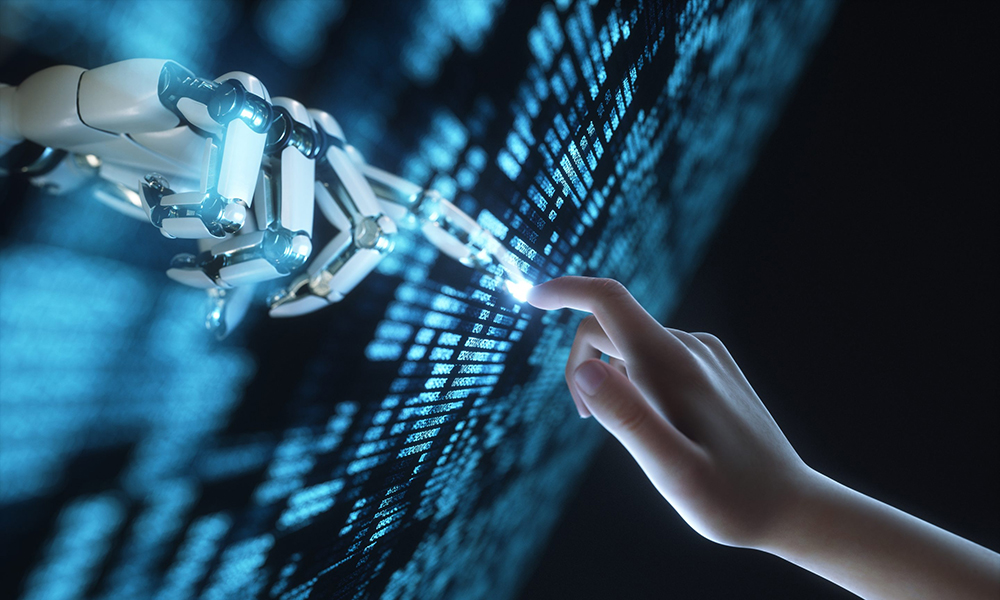
生成式人工智能首次亮相时,企业纷纷启动人工智能试验。人们接受了很多不太理解或许也不完全信任的创新。然而对网络安全专业人士来说,发挥人工智能的能力是多年宿愿,而且历史性的里程碑即将出现:预报攻击的能力。
在网络安全领域,事先预报一直是“至高核心”,但这一想法向来遭到质疑,而且确实有充分理由。有关“预报能力”的说法要么是营销炒作,要么就为时过早。然而,如今人工智能正处于转折点,更多数据访问,运转更流畅的模型以及数十年的经验积累为大规模预报铺平了道路。
看到这里,读者可能认为我马上就要暗示聊天机器人会演变成网络预言家,不会的,大伙可以松口气了。新一代聊天机器人使用的生成式人工智能尚未达到最佳性能。这只是开始,当前技术为基础模型和推理能力开辟道路,才能实现高度自信地判断网络攻击的可能性,具体攻击方式以及何时发生。
经典人工智能模型
想要短期内掌握基础模型可为安全团队带来的优势,必须首先了解这一领域人工智能发展的现状。经典人工智能模型利用针对特定用例的特定数据集训练,快速准确地获得特定结果,这是人工智能应用在网络安全中的关键优势。时至今日,相关创新加上自动化,在管理威胁、保护用户身份和数据隐私方面继续发挥着重要作用。
如果经典人工智能用Clop勒索软件(对数百个组织造成严重破坏的变体)训练,就能识别各种特征和细微线索,推断出环境中存在勒索软件,并优先标记提示安全团队。完成的速度和准确度都很高,明显超过手动分析。
今天,威胁模式出现了变化。攻击面逐渐扩大,对手跟企业一样使用人工智能,安全技能仍然稀缺。传统人工智能无法独立覆盖各种基础。
自我训练的人工智能模型
最近生成式人工智能兴起将大型语言模型(LLM)推向了网络安全领域的中心,因为大语言模型能利用自然语言为安全分析师快速获取和总结各种形式的信息。这些模型为安全团队提供类似人类的交互体验,使复杂且技术含量很高的信息消化和分析更方便也更迅速。
我们开始发现大语言模型能帮团队更快也更准确地做出决策。在某些情况下,以前需要数周的操作现在几天甚至数小时就能完成。当然,速度和准确性仍然是新型创新的关键特征。比较知名的案例包括IBM Watson Assistant、微软Copilot或Crowdstrike的Charlotte 人工智能聊天机器人等等技术突破。
安全市场当前的创新前沿在于:实现大语言模型的价值,主要途径是作为安全分析师人工助理的聊天机器人。未来12至18个月内,创新将转化应用并产生实质性影响。
由于行业人才短缺,安全人员每天面临的威胁也不断增加,各方面能争取到的帮助都需要,而且聊天机器人可将能量成倍放大。考虑一下,网络犯罪分子已将执行勒索软件攻击的时间减少94%:既然犯罪分子将时间当成武器,防守方也必须尽可能缩短应对时间。
然而,在基础模型对网络安全影响方面,网络聊天机器人只是前菜。
处于创新中心的基础模型
大语言模型成熟后,我们将能充分利用基础模型的潜力。基础模型可在多模式数据上训练——不仅包括文本,还有图像、音频、视频、网络数据、行为等。可在大语言模型简单语言处理基础上搭建,显著增加或替代人工智能当前需要的大量参数。再加上基础模型可自我监督,本身比较直观且适应性强。
具体什么意思?之前提到的勒索软件案例中,基础模型不必了解Clop勒索软件,甚至不用了解任何勒索软件,就能发现异常可疑的行为。基础模型可以自行学习,不需要针对特定的场景训练。因此在这种情况下,基础模型能发现难以捉摸的、前所未有的威胁。这一能力可提高安全分析师的效率,加快调查和响应。
相关能力距离实现已不远。大概一年前,我们在IBM启动了一个试验项目,为安全部门研发基础模型,监测之前无法发现的威胁并做出预报,在不损害数据隐私的前提下在企业安全堆栈中实现直观通信和推理。
客户一次试验中,该模型的新功能在攻击几天之前就预报了55次攻击。分析人士的证据显示,55个预报中23次攻击确实出现,其他多次攻击在被发现之前就已被阻止。其中包括多次分布式拒绝服务(DDoS),还有企图配置不同恶意软件的网络钓鱼攻击。提前了解对手的意图并为攻击做好准备,防守方就能掌握难得的富裕时间。
基础模型的训练数据来自几个相互影响的数据源——从API源、情报源、危害指标到行为和社交平台指标等。基础模型能帮我们 "发现 "对手利用客户环境中已知漏洞的意图,以及成功入侵后外泄数据的计划。此外,该模型假设了300多种新攻击模式,企业可利用相关信息强化安全防护。
相关知识给防守方争取到富裕时间的重要性不言而喻。了解即将到来的攻击后,安全团队可以采取应对措施,防止造成严重后果(例如,修补漏洞和纠正错误配置),也能为主动威胁类攻击做好准备。
如果说基础模型能阻止网络威胁,让全世界网络更安全,我会高兴得难以言喻,然而实际情况并不一定如此。预报并不是预言,而是经过证实的预测。(财富中文网)
斯里达尔·穆皮迪(Sridhar Muppidi)是IBM研究员,也是IBM Security首席技术官。
译者:梁宇
审校:夏林
生成式人工智能首次亮相时,企业纷纷启动人工智能试验。人们接受了很多不太理解或许也不完全信任的创新。然而对网络安全专业人士来说,发挥人工智能的能力是多年宿愿,而且历史性的里程碑即将出现:预报攻击的能力。
在网络安全领域,事先预报一直是“至高核心”,但这一想法向来遭到质疑,而且确实有充分理由。有关“预报能力”的说法要么是营销炒作,要么就为时过早。然而,如今人工智能正处于转折点,更多数据访问,运转更流畅的模型以及数十年的经验积累为大规模预报铺平了道路。
看到这里,读者可能认为我马上就要暗示聊天机器人会演变成网络预言家,不会的,大伙可以松口气了。新一代聊天机器人使用的生成式人工智能尚未达到最佳性能。这只是开始,当前技术为基础模型和推理能力开辟道路,才能实现高度自信地判断网络攻击的可能性,具体攻击方式以及何时发生。
经典人工智能模型
想要短期内掌握基础模型可为安全团队带来的优势,必须首先了解这一领域人工智能发展的现状。经典人工智能模型利用针对特定用例的特定数据集训练,快速准确地获得特定结果,这是人工智能应用在网络安全中的关键优势。时至今日,相关创新加上自动化,在管理威胁、保护用户身份和数据隐私方面继续发挥着重要作用。
如果经典人工智能用Clop勒索软件(对数百个组织造成严重破坏的变体)训练,就能识别各种特征和细微线索,推断出环境中存在勒索软件,并优先标记提示安全团队。完成的速度和准确度都很高,明显超过手动分析。
今天,威胁模式出现了变化。攻击面逐渐扩大,对手跟企业一样使用人工智能,安全技能仍然稀缺。传统人工智能无法独立覆盖各种基础。
自我训练的人工智能模型
最近生成式人工智能兴起将大型语言模型(LLM)推向了网络安全领域的中心,因为大语言模型能利用自然语言为安全分析师快速获取和总结各种形式的信息。这些模型为安全团队提供类似人类的交互体验,使复杂且技术含量很高的信息消化和分析更方便也更迅速。
我们开始发现大语言模型能帮团队更快也更准确地做出决策。在某些情况下,以前需要数周的操作现在几天甚至数小时就能完成。当然,速度和准确性仍然是新型创新的关键特征。比较知名的案例包括IBM Watson Assistant、微软Copilot或Crowdstrike的Charlotte 人工智能聊天机器人等等技术突破。
安全市场当前的创新前沿在于:实现大语言模型的价值,主要途径是作为安全分析师人工助理的聊天机器人。未来12至18个月内,创新将转化应用并产生实质性影响。
由于行业人才短缺,安全人员每天面临的威胁也不断增加,各方面能争取到的帮助都需要,而且聊天机器人可将能量成倍放大。考虑一下,网络犯罪分子已将执行勒索软件攻击的时间减少94%:既然犯罪分子将时间当成武器,防守方也必须尽可能缩短应对时间。
然而,在基础模型对网络安全影响方面,网络聊天机器人只是前菜。
处于创新中心的基础模型
大语言模型成熟后,我们将能充分利用基础模型的潜力。基础模型可在多模式数据上训练——不仅包括文本,还有图像、音频、视频、网络数据、行为等。可在大语言模型简单语言处理基础上搭建,显著增加或替代人工智能当前需要的大量参数。再加上基础模型可自我监督,本身比较直观且适应性强。
具体什么意思?之前提到的勒索软件案例中,基础模型不必了解Clop勒索软件,甚至不用了解任何勒索软件,就能发现异常可疑的行为。基础模型可以自行学习,不需要针对特定的场景训练。因此在这种情况下,基础模型能发现难以捉摸的、前所未有的威胁。这一能力可提高安全分析师的效率,加快调查和响应。
相关能力距离实现已不远。大概一年前,我们在IBM启动了一个试验项目,为安全部门研发基础模型,监测之前无法发现的威胁并做出预报,在不损害数据隐私的前提下在企业安全堆栈中实现直观通信和推理。
客户一次试验中,该模型的新功能在攻击几天之前就预报了55次攻击。分析人士的证据显示,55个预报中23次攻击确实出现,其他多次攻击在被发现之前就已被阻止。其中包括多次分布式拒绝服务(DDoS),还有企图配置不同恶意软件的网络钓鱼攻击。提前了解对手的意图并为攻击做好准备,防守方就能掌握难得的富裕时间。
基础模型的训练数据来自几个相互影响的数据源——从API源、情报源、危害指标到行为和社交平台指标等。基础模型能帮我们 "发现 "对手利用客户环境中已知漏洞的意图,以及成功入侵后外泄数据的计划。此外,该模型假设了300多种新攻击模式,企业可利用相关信息强化安全防护。
相关知识给防守方争取到富裕时间的重要性不言而喻。了解即将到来的攻击后,安全团队可以采取应对措施,防止造成严重后果(例如,修补漏洞和纠正错误配置),也能为主动威胁类攻击做好准备。
如果说基础模型能阻止网络威胁,让全世界网络更安全,我会高兴得难以言喻,然而实际情况并不一定如此。预报并不是预言,而是经过证实的预测。(财富中文网)
斯里达尔·穆皮迪(Sridhar Muppidi)是IBM研究员,也是IBM Security首席技术官。
译者:梁宇
审校:夏林
When generative AI made its debut, businesses entered an AI experiment. They bought in on innovations that many of them don’t quite understand or, perhaps, fully trust. However, for cybersecurity professionals, harnessing the potential of AI has been the vision for years–and a historic milestone will soon be reached: the ability to predict attacks.
The idea of predicting anything has always been the “holy grail” in cybersecurity, and one met, for good reason, with significant skepticism. Any claim about “predictive capabilities” has turned out to be either marketing hype or a premature aspiration. However, AI is now at an inflection point where access to more data, better-tuned models, and decades of experience have carved a more straightforward path toward achieving prediction at scale.
By now, you might think I’m a few seconds away from suggesting chatbots will morph into cyber oracles, but no, you can sigh in relief. Generative AI has not reached its peak with next-gen chatbots. They’re only the beginning, blazing a trail for foundation models and their reasoning ability to evaluate with high confidence the likelihood of a cyberattack, and how and when it will occur.
Classical AI models
To grasp the advantage that foundation models can deliver to security teams in the near term, we must first understand the current state of AI in the field. Classical AI models are trained on specific data sets for specific use cases to drive specific outcomes with speed and precision, the key advantages of AI applications in cybersecurity. And to this day, these innovations, coupled with automation, continue to play a drastic role in managing threats and protecting users’ identity and data privacy.
With classical AI, if a model was trained on Clop ransomware (a variant that has wreaked havoc on hundreds of organizations), it would be able to identify various signatures and subtleties inferring that this ransomware is in your environment and flag it with priority to the security team. And it would do it with exceptional speed and precision that surpasses manual analysis.
Today, the threat model has changed. The attack surface is expanding, adversaries are leaning on AI just as much as enterprises are, and security skills are still scarce. Classical AI cannot cover all bases on its own.
Self-trained AI models
The recent boom of generative AI pushed Large Language Models (LLMs) to centerstage in the cybersecurity sector because of their ability to quickly fetch and summarize various forms of information for security analysts using natural language. These models deliver human-like interaction to security teams, making the digestion and analysis of complex, highly technical information significantly more accessible and much quicker.
We’re starting to see LLMs empower teams to make decisions faster and with greater accuracy. In some instances, actions that previously required weeks are now completed in days–and even hours. Again, speed and precision remain the critical characteristics of these recent innovations. Salient examples are breakthroughs introduced with IBM Watson Assistant, Microsoft Copilot, or Crowdstrike’s Charlotte AI chatbots.
In the security market, this is where innovation is right now: materializing the value of LLMs, mainly through chatbots positioned as artificial assistants to security analysts. We’ll see this innovation convert to adoption and drive material impact over the next 12 to 18 months.
Considering the industry talent shortage and rising volume of threats that security professionals face daily, they need all the helping hands they can get–and chatbots can act as a force multiplier there. Just consider that cybercriminals have been able to reduce the time required to execute a ransomware attack by 94%: they’re weaponizing time, making it essential for defenders to optimize their own time to the maximum extent possible.
However, cyber chatbots are just precursors to the impact that foundation models can have on cybersecurity.
Foundation models at the epicenter of innovation
The maturation of LLMs will allow us to harness the full potential of foundation models. Foundation models can be trained on multimodal data–not just text but image, audio, video, network data, behavior, and more. They can build on LLMs’ simple language processing and significantly augment or supersede the current volume of parameters that AI is bound to. Combined with their self-supervised nature, they become innately intuitive and adaptable.
What does this mean? In our previous ransomware example, a foundation model wouldn’t need to have ever seen Clop ransomware–or any ransomware for that matter–to pick up on anomalous, suspicious behavior. Foundation models are self-learning. They don’t need to be trained for a specific scenario. Therefore, in this case, they’d be able to detect an elusive, never-before-seen threat. This ability will augment security analysts’ productivity and accelerate their investigation and response.
These capabilities are close to materializing. About a year ago, we began running a trial project at IBM, pioneering a foundation model for security to detect previously unseen threats, foresee them, and empower intuitive communication and reasoning across an enterprise’s security stack without compromising data privacy.
In a client trial, the model’s nascent capabilities predicted 55 attacks several days before the attacks even occurred. Of those 55 predictions, the analysts have evidence that 23 of those attempts took place as expected, while many of the other attempts were blocked before they hit the radar. Amongst others, this included multiple Distributed Denial of Service (DDoS) attempts and phishing attacks intending to deploy different malware strains. Knowing adversaries’ intentions ahead of time and prepping for these attempts gave defenders a time surplus they don’t often.
The training data for this foundation model comes from several data sources that can interact with each other–from API feeds, intelligence feeds, and indicators of compromise to indicators of behavior and social platforms, etc. The foundation model allowed us to “see” adversaries’ intention to exploit known vulnerabilities in the client environment and their plans to exfiltrate data upon a successful compromise. Additionally, the model hypothesized over 300 new attack patterns, which is information organizations can use to harden their security posture.
The importance of the time surplus this knowledge gave defenders cannot be overstated. By knowing what specific attacks were coming, our security team could run mitigation actions to stop them from achieving impact (e.g., patching a vulnerability and correcting misconfigurations) and prepare its response for those manifesting into active threats.
While it would bring me no greater joy than to say foundation models will stop cyber threats and render the world cyber-secure, that’s not necessarily the case. Predictions aren’t prophecies–they are substantiated forecasts.
Sridhar Muppidi is an IBM fellow and CTO of IBM Security.